Deploying artificial intelligence (AI) will bring up added security issues that organisations need to address, such as potential bias in AI models, or risk seeing their investments go to waste.
In fact, the rush to adopt AI has resulted in some businesses overlooking the need to do so responsibly.
More than 80% of respondents in a global survey by NTT Data revealed that their leadership, governance, and workforce readiness were failing to keep up with AI advancements.
Just 24% of CISOs believed their organisation had a strong framework that balanced AI risks and value creation, despite 89% of C-suite leaders worrying about AI security risks.
Companies are divided internally with a desire to drive ahead with innovation while also wanting to manage the risks and uncertainties around AI, said John Lombard, Asia-Pacific CEO for NTT Data.
He noted that there were different perspectives in the boardroom, where there also were concerns around the lack of clarity in terms of AI regulations.
More than 80% noted that this was hindering AI investment and implementation, leading to delayed adoption, revealed the NTT Data study. Another 89% cited AI security risks as a real concern, with just 24% noting that their security posture was strong enough to support an AI environment.
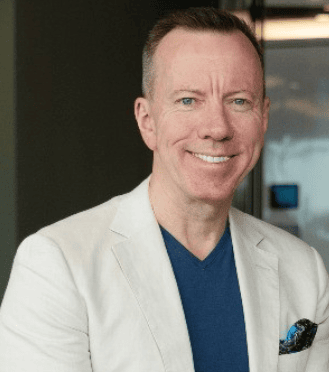
Bringing in AI technologies creates additional security risks, such as potential misuse and data leaks, that need to be addressed, Lombard said in a video interview with FutureCISO. There also is added pressure on the underlying data infrastructure, with organisations pulling from different data sources and potentially exposing their corporate data in an undesired environment, he said.
These challenges can slow down adoption, he added, noting that the board’s role is to govern the company’s AI strategy and direction. A lack of alignment in the boardroom can slow down adoption of AI, as the NTT Data study indicates, he said.
Lombard stressed the need for organisations to stay on top of such issues and establish a framework that facilitates strong governance and responsible use.
This goes beyond a typical digital transformation roadmap and encompasses several areas, such as ethical considerations, data privacy, transparency, sustainability, and the impact on employees and how they work, he said.
Organisations looking at AI adoption often are most concerned about privacy and how their data will be used, noted Robert Young, vice president of strategic innovation at Aicadium, an AI technology vendor founded by Singapore-owned investment firm Temasek Holdings. Aicadium focuses on AI-powered industrial computer vision applications.
IT governance should include AI considerations
They want to know if the data use complies with their industry’s data privacy guidelines and internal IT governance, Young told FutureCISO in a video interview, adding that these often are companies from highly regulated industries, such as financial services.
“So we’re seeing an expansion of IT governance to include AI and how data is managed,” he said. “There often are questions [from organisations] around how models are trained and the safeguards around how they’re used.”
The team tasked to implement AI tools often are also responsible in ensuring these are used for their intended purpose, he noted.
There is added focus on data ownership, especially related to LLMs (large language models), including discussions around who can train these AI models and who owns the data, said Phoebe Poon, vice president of product management at Aicadium.
In Asia-Pacific particularly, companies will want to know if the AI models understand context in the languages relevant to markets in the region, Poon said.
AI initiatives should not be treated as a typical IT project, as the issues around the technology are unique, Lombard said.
Companies also will need to determine if they have the relevant skillsets and to plug the gaps, if any, he added.
“Without clear leadership, the responsibility gap threatens to derail responsible AI and GenAI (generative AI) development, letting investment in this area go to waste and stifling progress from experimentation to implementation,” he said. “Companies in Asia-Pacific must first define what responsibility means to their organisation and align it with their mission, visions, and values.”
“The enthusiasm for AI is undeniable, but our findings show that innovation without responsibility is a risk multiplier,” NTT Data CEO Abhijit Dubey said in the study. “Organisations need leadership-driven AI governance strategies to close this gap, before progress stalls and trust erodes.”
Addressing potential bias in AI models
Companies also will need to be mindful about potential bias in AI models, in particular, if they are applying these to support services in specific regions or markets.
Research from Singapore’s Infocomm Media Development Authority (IMDA) uncovered bias in major LLMs across various areas, such as gender and culture. Tests, for instance, found that model guardrails for cultural biases in non-English languages might not hold up as well as those in English. Regional language prompts had a higher percentage, at 69.4%, of successful exploits – responses deemed to be biased -- compared to 30.6% for English language prompts.
The findings indicate the extent to which AI model safety for non-English language systems lag behind their English counterparts.
Researchers in the IMDA exercise also found that gender bias clocked the highest percentage of successful exploits, at 26.1%, followed by race, religious, and ethnic bias at 22.8% and geographical identity bias at 22.6%.
As it is, 65% of business leaders in Asia-Pacific acknowledged that key business decisions were based on inaccurate or inconsistent data most of the time, if not always, according to a SoftServ study released in February. This figure was higher than the global average of 65%.
Another 77% of Asia-Pacific respondents believed no one in their organisation understood all the data collected and how to access it, compared to the global average of 58%.
To help address AI bias, IMDA and its subsidiary AI Verify Foundation in February announced plans for a Global AI Assurance Pilot, which they said would provide norms and best practices around technical testing of GenAI applications. It focuses on technical testing of real-world applications, such as healthcare, finance, and public services.
For instance, technical testing of individual LLM applications could span various dimensions, including safety and health risks, lack of transparency, inappropriate data disclosure, and unfair treatment of staff and customers, IMDA said.
The pilot is slated to be complete by May this year.
Commenting on AI safety and testing, Singapore’s Minister for Digital Development and Information Josephine Teo said in February: “As we see more AI applications being brought to the market, the risks are becoming more prominent. For example, in the finance sector, we see more and more of the use of AI for credit approvals. The question is, if you are a bank customer, how can you be assured that this is not tilted against you and there isn’t some bias that is built in that makes it harder for you? That is when you need a proper process for robust testing for fairness.”
Through the Global AI Assurance Pilot, Teo said, Singapore hoped to provide a platform on which the private sector and government could work together to build trust in AI tools and offer guidelines on how AI should be implemented in a real-world setting.
Lombard also suggested the use of small- and medium-sized LLMs.
He pointed to industry-focus solutions trained on these smaller LLMs, which have been seeing growing interest among enterprises.
LLMs that better capture the language and culture of a specific group, for instance, might be a better fit than massive foundation models for some companies, he said.
He further stressed that LLMs are just one part of the solution, with the tools that sit alongside AI model just as important. These should enable businesses, among other functions, to build industry-specific use cases, decide how data should be utilised, and manage data security as well as access to data, he said.
Young also highlighted opportunities for further education, noting that removing bias from every AI model for every use case will be a long endeavour.
He underscored the importance of understanding what to look out for and to constantly assess AI models for potential bias, that is aligned with the company’s regional needs.
Noting that there can never be a “perfect” AI model that caters to every cultural environment, he highlighted the need to look at the use of AI and establish the safeguards around it to ensure people’s wellbeing.
Poon concurred: “AI model isn’t perfect, so awareness is important.”