Artificial intelligence has been immortalised in movies with hits like "Bicentennial Man", "Upgrade" and its namesake "Artificial Intelligence" by Steven Spielberg.
These days AI is getting a revival in the enterprise following OpenAI's launch of Chat Generative Pre-trainer Transformer on 30 November 2022. The large language model-based chatbot, more popularly known as ChatGPT, is making a lot of buzz in the boardroom, at conferences and almost everywhere imaginable.
Opportunities and risks
In a 2023 survey of 400 executives across Australia, Germany, India, Singapore, the UK and the USA, 64% of CEOs say they face significant pressure from investors, creditors, and lenders to accelerate adoption of Generative AI (GenAI).
The report, a collaboration between Oxford Economics and the IBM Institute of Business Value (IBV), noted that compared to what was understood about artificial intelligence in 2016 versus GenAI in 2023, executives have a much more focused view of where to deploy generative AI. They are much clearer on which use cases and AI applications they see as driving the most value (see Figure 1).
Figure 1: Three priorities for GenAI adoption
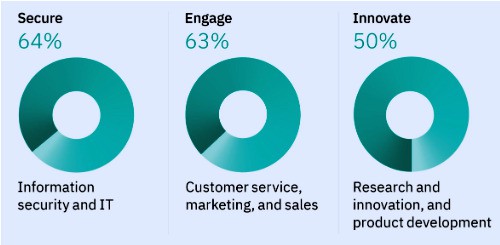
But as with other emerging technologies, generative AI carries as many risks as it offers opportunities. Sanjay Deshmukh, senior regional vice president for ASEAN and India at Snowflake acknowledged that GenAI democratised AI and many organisations are prioritising and embracing it.
He cautioned that companies need to understand their AI and data strategies as well as consider the business use cases and outcomes to fully leverage GenAI today. “It is the data that powers AI. Educating enterprises focusing on the business outcome is key to business success,” he continued.
What are the pros and cons of Generative AI?
Sanjay Deshmukh: There are no negatives to the technology. It is one of the most powerful innovations that will disrupt many industries; companies can derive business value from AI. ChatGPT and other fundamental models are trained based on external data in the public domain, thus it doesn’t have any real understanding of business and data such as data fraud. There is a possibility that my data might be used by the model to train, and will probably land with somebody else's responses.
Using your solution for the LMM model so the data stays inside to mitigate the risk of somebody accessing the data for their purposes or even accessing the data for nefarious reasons. This will require sizable computer and storage resources. Will it cost you a lot to build the infrastructure that will enable you to support the computer requirements of this data warehouse?
Sanjay Deshmukh: We are a firm believer in empowering organisations to mobilise data and build a single source of truth. Data silos challenge organizations; a unified platform is vital for simplification. What is scalable for organizations are computing and storage, also, organisations can use a single-purpose model through a targeted model using structured data from a set of documents. However, other models will need to be trained on an organisation's data and will need GPU with lesser computing power and cost.
In many parts of Asia, especially when it involves heavily regulated markets and governments have introduced data sovereignty issues. How do you get around the issue of data sovereignty if the Data is in the Snowflake Cloud?
Sanjay Deshmukh: Regardless of whether the data is inside the country parameter or not, as long as it is in the Snowflake environment, it is secure. The data is encrypted, and organisations can decide the level of security needed through masking or tokenising the data. Organisations such as those in the Philippines must give confidence to their regulators that they will meet the regulatory security requirements even though the data is hosted overseas in Singapore.
For leaders tasked with supervising the adoption of Gen AI, how should they balance their desires for innovations? What are your recommendations?
Sanjay Deshmukh: Without a data strategy, there is no AI strategy. Once the data is in one place, companies can leverage it to power AI-driven applications. The most common use case we observe is user productivity using a natural language query. Organisations need to build a security framework to classify which data needs to be protected. For the data that is not sensitive, democratise access to everyone so they can use it to make decisions.
Click on the PodChat player and listen to Deshmukh explain how to secure Generative AI for enterprises.
- What are the top 3 pros and cons of letting loose Generative AI in the enterprise?
- Speaking of security, given that GenAI is still evolving, how can the CIO/compliance officer mitigate the potential risks associated with this technology?
- Does your approach of taking the LLM to the data necessitate the need for a sizeable compute infrastructure on the part of the enduser organisation?
- How do you get around local/national data sovereignty regulations if the condition for an enduser to use your LLM technology is to have the customer's data reside in your platform – which could be outside the borders of the customer's country of operation?
- For leaders tasked with supervising the adoption of GenAI, how should they balance the desires for innovation, vis-à-vis the opportunities and risks that come with something that is still evolving?