We can think of generative AI as behaving like antibiotics: wonder drugs at their debut that became increasingly problematic over time as resistance built up, until they stop working altogether
Jason Bloomberg
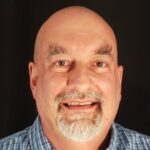
Jason Bloomberg, managing partner at IIntellyx explains some drawbacks of Artificial Intelligence (AI) despite its current prevalence.
The first one, Bloomberg says, is model collapse which “occurs when AI models train on AI-generated content. It’s a process where small errors or biases in generated data compound with each cycle, eventually steering the model away from generating inferences based on the original distribution of data. In other words, the model eventually forgets the original data entirely and ends up creating useless noise.”
The second major drawback of AI, according to Bloomberg, is data poisoning, which is considered a new threat to the technology. Nary Simms of La Salle University cited a recent survey among industrial practitioners where data poisoning is found to be the number one concern among threats to AI.
"Since defensive methods haven’t been tested under typical or real-world conditions, it’s not known how dangerous data poisoning is and which methods work."
Nary Simms
Data Poisoning, a major threat
Ian Lim, field chief security officer, JAPAC, Palo Alto Networks considers data poisoning as a tactic of sophisticated attackers and it is used to disrupt the machine learning (ML) procedure by injecting a fraction of malicious samples into the training dataset.
“Attackers may infiltrate a system to take control or change the behaviour of the system by tampering with the training data using false data, which ML then processes. As a result, the reliability of the system may be compromised, which poses risks to various security-critical domains of the system,” Lim says.
Orchestration and repercussion
Lim notes that AI and ML use in the cybersecurity space has increased significantly in recent years, particularly in predictive analytics to detect signatures of new malware attacking a system.
He explains that instead of an external attack, threat actors may attempt to poison the private training data of an ML model, affecting the accuracy of the model’s predictions and detection system.
“This aligns with Palo Alto Networks’ prediction that threat actors always seek more advanced techniques to evade security detection and for vulnerable systems to infiltrate,” Lim explains.
“Our Unit 42 2023 Network Threat Trends Report Vol. 2 also outlined that vulnerability exploitation shows no sign of slowing down – in 2022 Unit 42 found 228,000 attempts, an increase from 147,000 exploitation attempts in 2022. The report found that vulnerabilities that are disclosed and not yet disclosed are both at risk to be exploited by threat actors.”
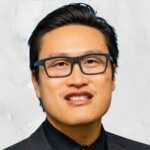
The Challenge
“The explosion of IoT devices has led to massive amounts of data available. As data becomes more vast and heterogeneous, the features of data are also more complex to understand. This means that attackers have more chances to manipulate the data collected from various sources.”
Ian Lim
Even a small amount of compromised data causes significant damage to ML systems, which makes it almost impossible to validate and curate the data. Lim says data poisoning is relatively easy to execute because there is no need for high-computational devices or a lot of information about the data set.
"With large amounts of data available and being processed constantly, spotting poisoned data is challenging and requires a lot of time. This is because the process involves analysing all inputs against a set of multiple classifiers before retraining the sanitized model."
Ian Lim
Lim says there are a lot of ways data poisoning infiltrates tools defenders used by ML models such as modification of special input data to evade intrusion detection systems to reach internal systems, injecting poisoned and misleading samples directly into the training dataset to change the behaviour of the malware detection system, and the process called crowdturfing or “creating large amounts of user accounts with false data to mislead the classifier of ML, which then modifies the ML algorithms,” Lim explains.
Protective measures
The Palo Alto Networks officer advises the implementation of the following measures for organisations in the cybersecurity space using AI/ML detection systems
- Data sanitization – conducted by separating and removing malicious samples from normal ones. Any changes in the characteristics of the training data are detected or identified. Moreover, any outliers that are suspected to be malicious are removed.
- De-Pois – an attack-agnostic approach in which a mimic model imitating the target model behaviour is constructed. This will allow straightforward identification of poisoned samples from the clean ones through comparisons of prediction differences.
- Developing AI models to regularly check that all the labels in their training data are accurate
- Pentesting – using simulated cyberattacks to expose gaps and liabilities
- Some researchers also suggest adding a second layer of AI and ML to catch potential errors in the training dataset
The OWASP Foundation, further notes that organisations should invest in data validation, verification, storage security, and separation, as well as limiting access to training data. Models should also be validated to detect any poisoning attacks and multiple models should be trained through different subsets. Furthermore, anomaly detection techniques should be deployed to detect any abnormality in training data.
Zero trust
Moreover, Lim says organisations need to be vigilant and find new ways to get ahead of threat actors. Organisations can implement Zero Trust measures to protect the integrity of the AI/ML environments. “This means assuming a hostile environment and designing defence-in-depth into every layer of the organisation,” Lim says.
Zero Trust principles enforce thorough inspection and continuous validation of all digital interactions (users and machines) and have the ability to quickly respond to cyber attacks by leveraging automation and training SOC analysts to look for sophisticated attacks continually.